What is Regression Analysis?
Twingate Team
•
Jul 17, 2024
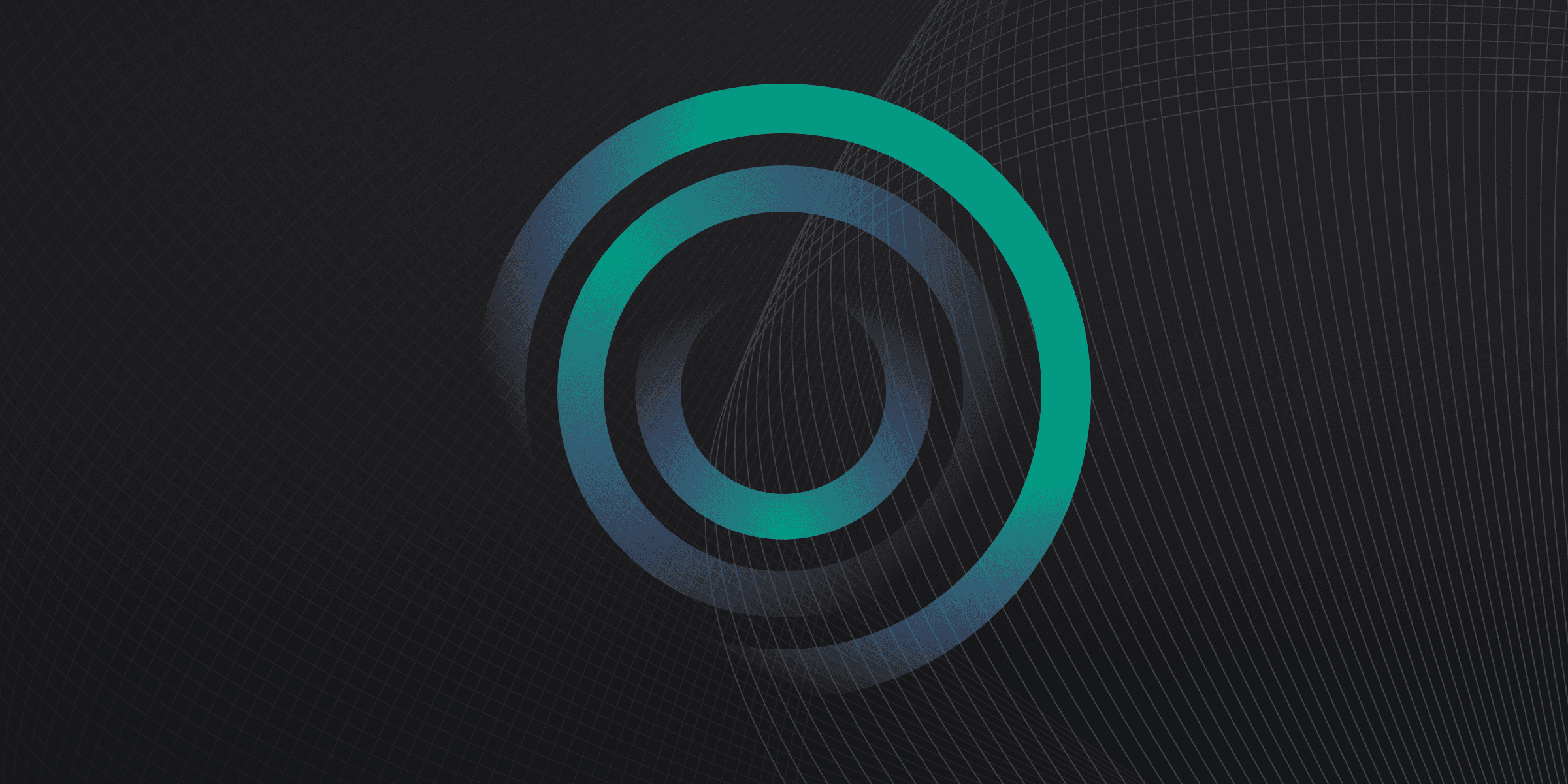
Regression analysis is a statistical method for estimating relationships among variables, focusing on the relationship between a dependent variable and one or more independent variables. It's used in various sectors, such as sales forecasting, real estate pricing, and health expenditure prediction, to understand trends and make strategic decisions based on historical data. However, it has limitations, including sensitivity to outliers and assumptions of linear relationships between variables.
Types of Regression Analysis
There are various types of regression analysis, each with its unique benefits and limitations. Choosing the appropriate type depends on the data, the objective of the analysis, and the relationship between variables. Some common types of regression analysis include:
Linear Regression: Predicts a continuous dependent variable based on one or more independent variables.
Logistic Regression: Predicts the probability of an event occurring based on one or more independent variables.
Polynomial Regression: Models the relationship between a dependent variable and independent variables using a polynomial function.
Ridge Regression: Addresses multicollinearity issues in multiple regression by adding a penalty term to the loss function.
Applications in Cybersecurity
Regression analysis has found its way into the realm of cybersecurity, helping organizations predict and mitigate potential threats. By analyzing historical data, cybersecurity professionals can identify trends and make informed decisions to protect their networks and systems. Some applications of regression analysis in cybersecurity include:
Threat Prediction: Identifying patterns in past cyber attacks to predict future threats and vulnerabilities.
Resource Allocation: Optimizing the distribution of cybersecurity resources based on the analysis of past incidents and their impact.
Security Measure Effectiveness: Evaluating the success of implemented security measures by analyzing their impact on reducing cyber threats.
Incident Response Planning: Using regression analysis to develop data-driven strategies for responding to cyber attacks and minimizing their impact.
Regression vs. Correlation: Understanding the Difference
Understanding the difference between regression and correlation is crucial for accurate data analysis and interpretation. Here are two main distinctions:
Regression: Predicts the outcome of a dependent variable based on one or more independent variables, focusing on the impact of changes in independent variables on the dependent variable.
Correlation: Measures the strength and direction of a linear relationship between two continuous variables, without implying causation.
Challenges in Implementing Regression Analysis
Implementing regression analysis in cybersecurity comes with its challenges, such as:
Data Quality: Ensuring the accuracy and relevance of the data used for analysis.
Variable Selection: Choosing appropriate independent variables for reliable predictions.
Result Interpretation: Correctly interpreting the outcomes for effective decision-making.
Rapidly implement a modern Zero Trust network that is more secure and maintainable than VPNs.
What is Regression Analysis?
Twingate Team
•
Jul 17, 2024
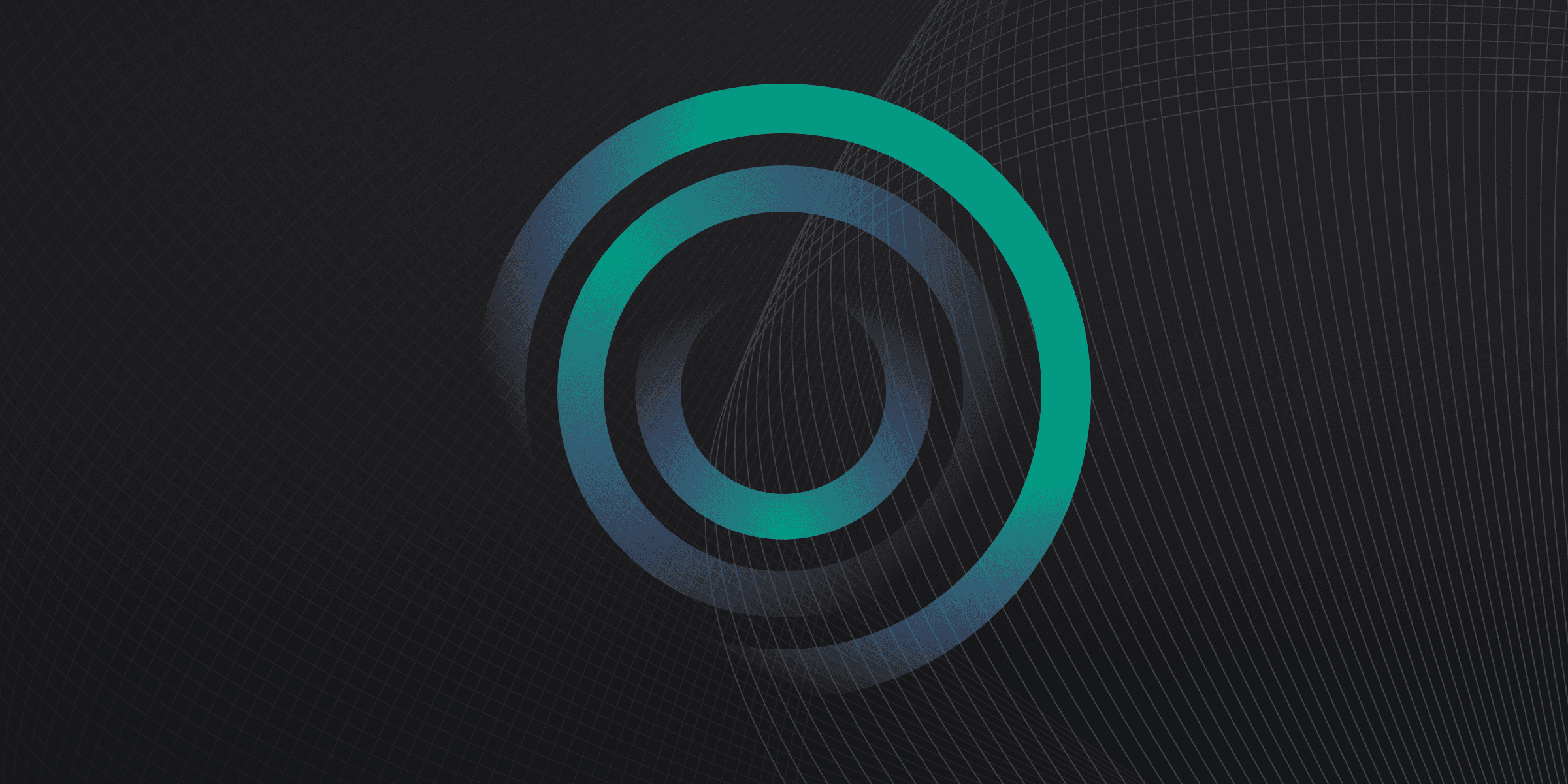
Regression analysis is a statistical method for estimating relationships among variables, focusing on the relationship between a dependent variable and one or more independent variables. It's used in various sectors, such as sales forecasting, real estate pricing, and health expenditure prediction, to understand trends and make strategic decisions based on historical data. However, it has limitations, including sensitivity to outliers and assumptions of linear relationships between variables.
Types of Regression Analysis
There are various types of regression analysis, each with its unique benefits and limitations. Choosing the appropriate type depends on the data, the objective of the analysis, and the relationship between variables. Some common types of regression analysis include:
Linear Regression: Predicts a continuous dependent variable based on one or more independent variables.
Logistic Regression: Predicts the probability of an event occurring based on one or more independent variables.
Polynomial Regression: Models the relationship between a dependent variable and independent variables using a polynomial function.
Ridge Regression: Addresses multicollinearity issues in multiple regression by adding a penalty term to the loss function.
Applications in Cybersecurity
Regression analysis has found its way into the realm of cybersecurity, helping organizations predict and mitigate potential threats. By analyzing historical data, cybersecurity professionals can identify trends and make informed decisions to protect their networks and systems. Some applications of regression analysis in cybersecurity include:
Threat Prediction: Identifying patterns in past cyber attacks to predict future threats and vulnerabilities.
Resource Allocation: Optimizing the distribution of cybersecurity resources based on the analysis of past incidents and their impact.
Security Measure Effectiveness: Evaluating the success of implemented security measures by analyzing their impact on reducing cyber threats.
Incident Response Planning: Using regression analysis to develop data-driven strategies for responding to cyber attacks and minimizing their impact.
Regression vs. Correlation: Understanding the Difference
Understanding the difference between regression and correlation is crucial for accurate data analysis and interpretation. Here are two main distinctions:
Regression: Predicts the outcome of a dependent variable based on one or more independent variables, focusing on the impact of changes in independent variables on the dependent variable.
Correlation: Measures the strength and direction of a linear relationship between two continuous variables, without implying causation.
Challenges in Implementing Regression Analysis
Implementing regression analysis in cybersecurity comes with its challenges, such as:
Data Quality: Ensuring the accuracy and relevance of the data used for analysis.
Variable Selection: Choosing appropriate independent variables for reliable predictions.
Result Interpretation: Correctly interpreting the outcomes for effective decision-making.
Rapidly implement a modern Zero Trust network that is more secure and maintainable than VPNs.
What is Regression Analysis?
Twingate Team
•
Jul 17, 2024
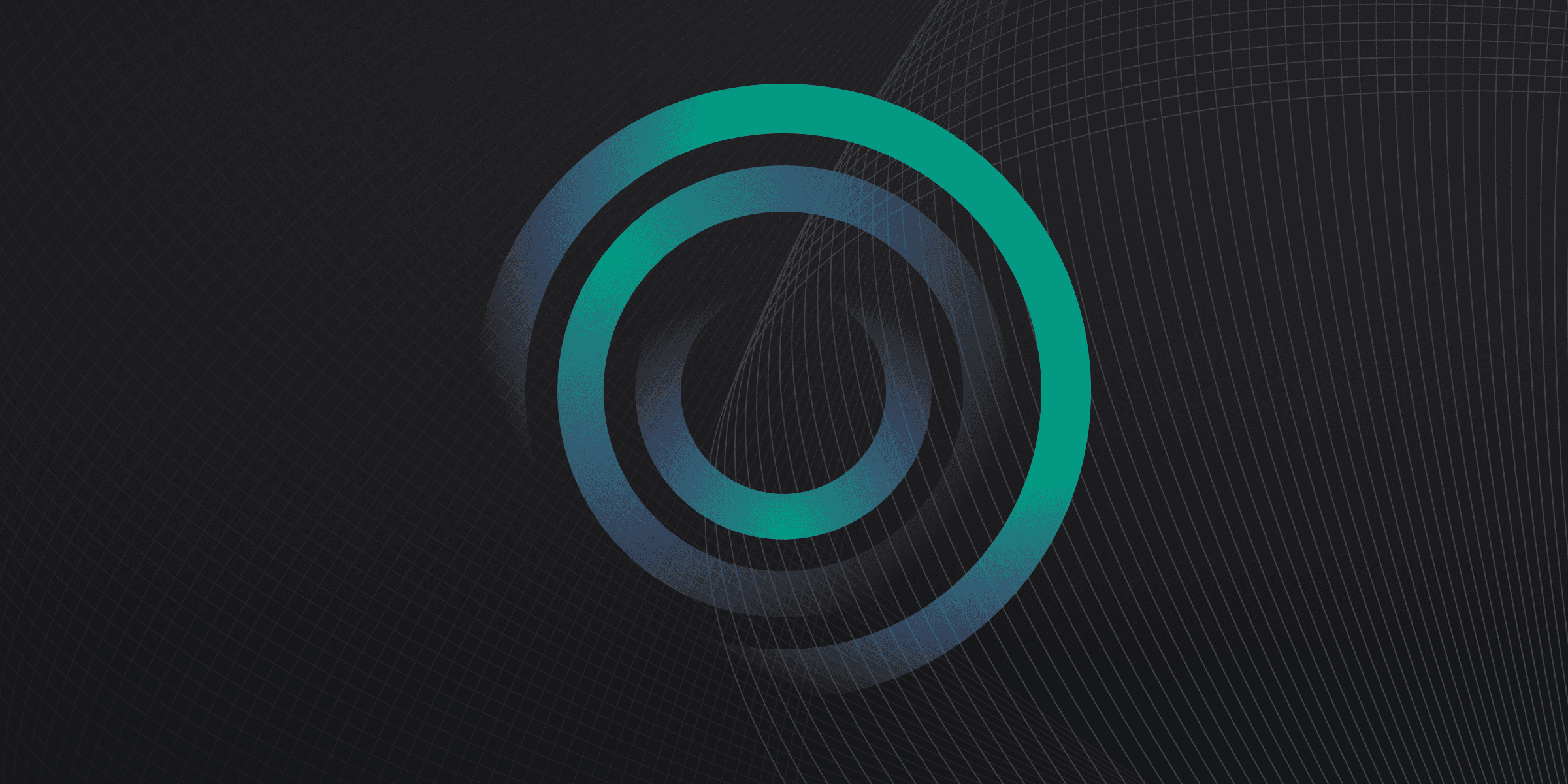
Regression analysis is a statistical method for estimating relationships among variables, focusing on the relationship between a dependent variable and one or more independent variables. It's used in various sectors, such as sales forecasting, real estate pricing, and health expenditure prediction, to understand trends and make strategic decisions based on historical data. However, it has limitations, including sensitivity to outliers and assumptions of linear relationships between variables.
Types of Regression Analysis
There are various types of regression analysis, each with its unique benefits and limitations. Choosing the appropriate type depends on the data, the objective of the analysis, and the relationship between variables. Some common types of regression analysis include:
Linear Regression: Predicts a continuous dependent variable based on one or more independent variables.
Logistic Regression: Predicts the probability of an event occurring based on one or more independent variables.
Polynomial Regression: Models the relationship between a dependent variable and independent variables using a polynomial function.
Ridge Regression: Addresses multicollinearity issues in multiple regression by adding a penalty term to the loss function.
Applications in Cybersecurity
Regression analysis has found its way into the realm of cybersecurity, helping organizations predict and mitigate potential threats. By analyzing historical data, cybersecurity professionals can identify trends and make informed decisions to protect their networks and systems. Some applications of regression analysis in cybersecurity include:
Threat Prediction: Identifying patterns in past cyber attacks to predict future threats and vulnerabilities.
Resource Allocation: Optimizing the distribution of cybersecurity resources based on the analysis of past incidents and their impact.
Security Measure Effectiveness: Evaluating the success of implemented security measures by analyzing their impact on reducing cyber threats.
Incident Response Planning: Using regression analysis to develop data-driven strategies for responding to cyber attacks and minimizing their impact.
Regression vs. Correlation: Understanding the Difference
Understanding the difference between regression and correlation is crucial for accurate data analysis and interpretation. Here are two main distinctions:
Regression: Predicts the outcome of a dependent variable based on one or more independent variables, focusing on the impact of changes in independent variables on the dependent variable.
Correlation: Measures the strength and direction of a linear relationship between two continuous variables, without implying causation.
Challenges in Implementing Regression Analysis
Implementing regression analysis in cybersecurity comes with its challenges, such as:
Data Quality: Ensuring the accuracy and relevance of the data used for analysis.
Variable Selection: Choosing appropriate independent variables for reliable predictions.
Result Interpretation: Correctly interpreting the outcomes for effective decision-making.
Solutions
Solutions
The VPN replacement your workforce will love.
Solutions