What is an Inference Attack?
Twingate Team
•
Oct 9, 2024
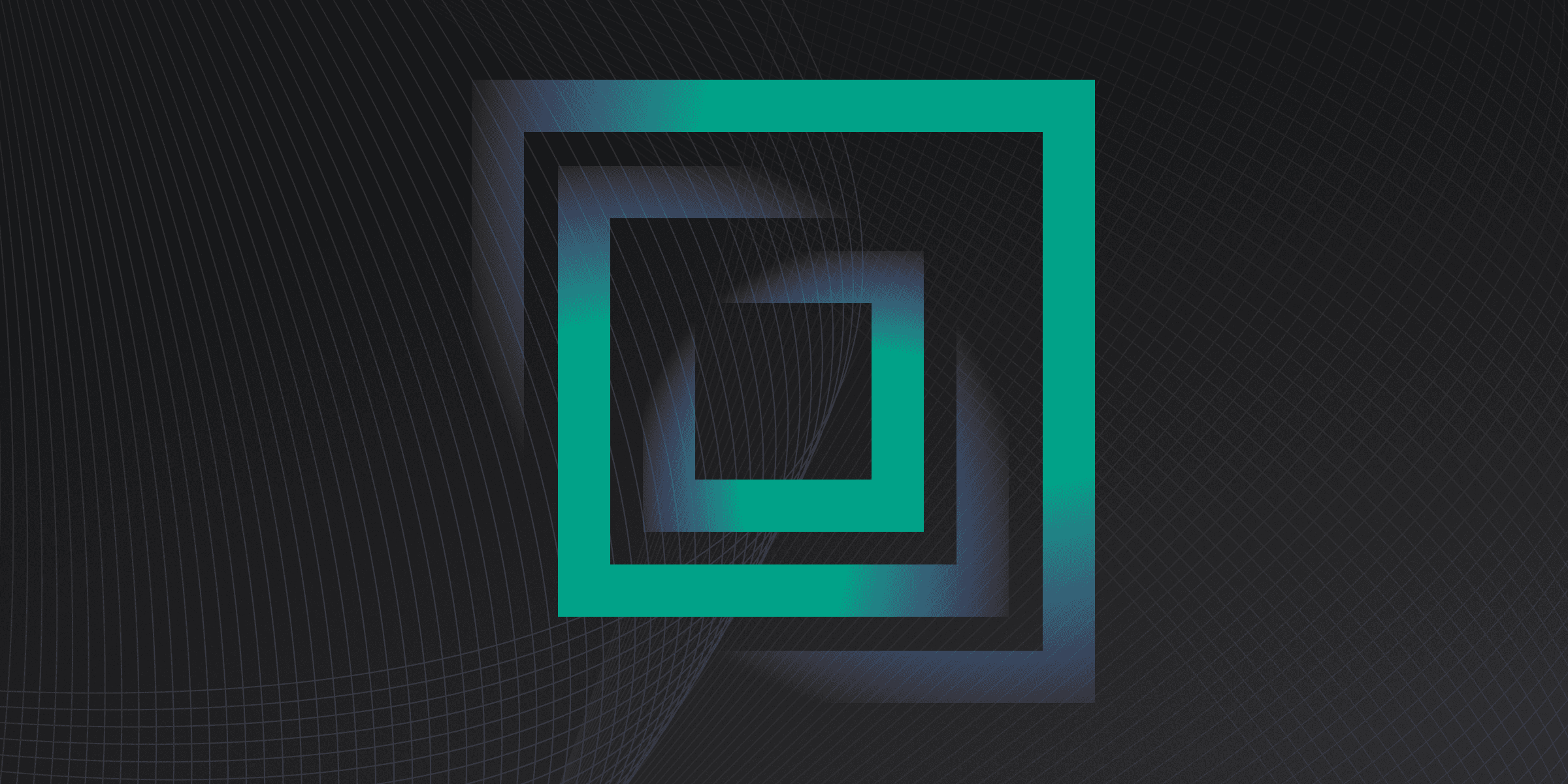
An inference attack is a data mining technique where attackers deduce sensitive information from seemingly unrelated data points.
Types of Inference Attacks
Inference attacks come in various forms, each exploiting different types of data to deduce sensitive information. Understanding these types can help in developing better security measures to protect against them.
Statistical Databases: Attackers analyze statistical data to infer individual records.
IoT Devices: Data from smart devices can reveal personal information about users.
Mobile Devices: Sensor data like accelerometers can infer user behavior and location.
Voice Recordings: Analyzing voice commands to extract sensitive information.
Recognizing Inference Attack Vulnerabilities
Recognizing inference attack vulnerabilities is crucial for safeguarding sensitive information. By understanding the various ways attackers can exploit data, organizations can better protect their assets and user privacy.
Statistical Data: Attackers analyze aggregated data to infer individual details.
IoT Devices: Poorly secured smart devices can leak personal information.
Mobile Sensors: Data from sensors like accelerometers can reveal user behavior.
Voice Data: Analyzing voice recordings to extract sensitive information.
Mitigating Inference Attacks Strategies
Mitigating inference attacks requires a multi-faceted approach to ensure data security and privacy. By implementing robust strategies, organizations can significantly reduce the risk of sensitive information being deduced from seemingly unrelated data points.
Polyinstantiation: Maintain multiple records with the same key to prevent inference.
Data Anonymization: Modify fields while preserving aggregate characteristics.
Defense In-Depth: Use multiple layers of security to guard against failures.
Least Privilege: Allow minimal permissions necessary for tasks.
Separation of Duties: Split privileges among individuals to prevent fraud.
Inference Attacks in the Wild
Inference attacks are not just theoretical; they have been observed in various real-world scenarios, demonstrating their potential to compromise sensitive information. Here are some notable examples of inference attacks in the wild:
Healthcare Data: Attackers deduced patient information from anonymized health records.
Social Media: Personal details inferred from public posts and interactions.
Smart Meters: Energy usage patterns revealing household activities.
Mobile Apps: User behavior inferred from app usage and sensor data.
Voice Assistants: Sensitive information extracted from voice command patterns.
Rapidly implement a modern Zero Trust network that is more secure and maintainable than VPNs.
What is an Inference Attack?
Twingate Team
•
Oct 9, 2024
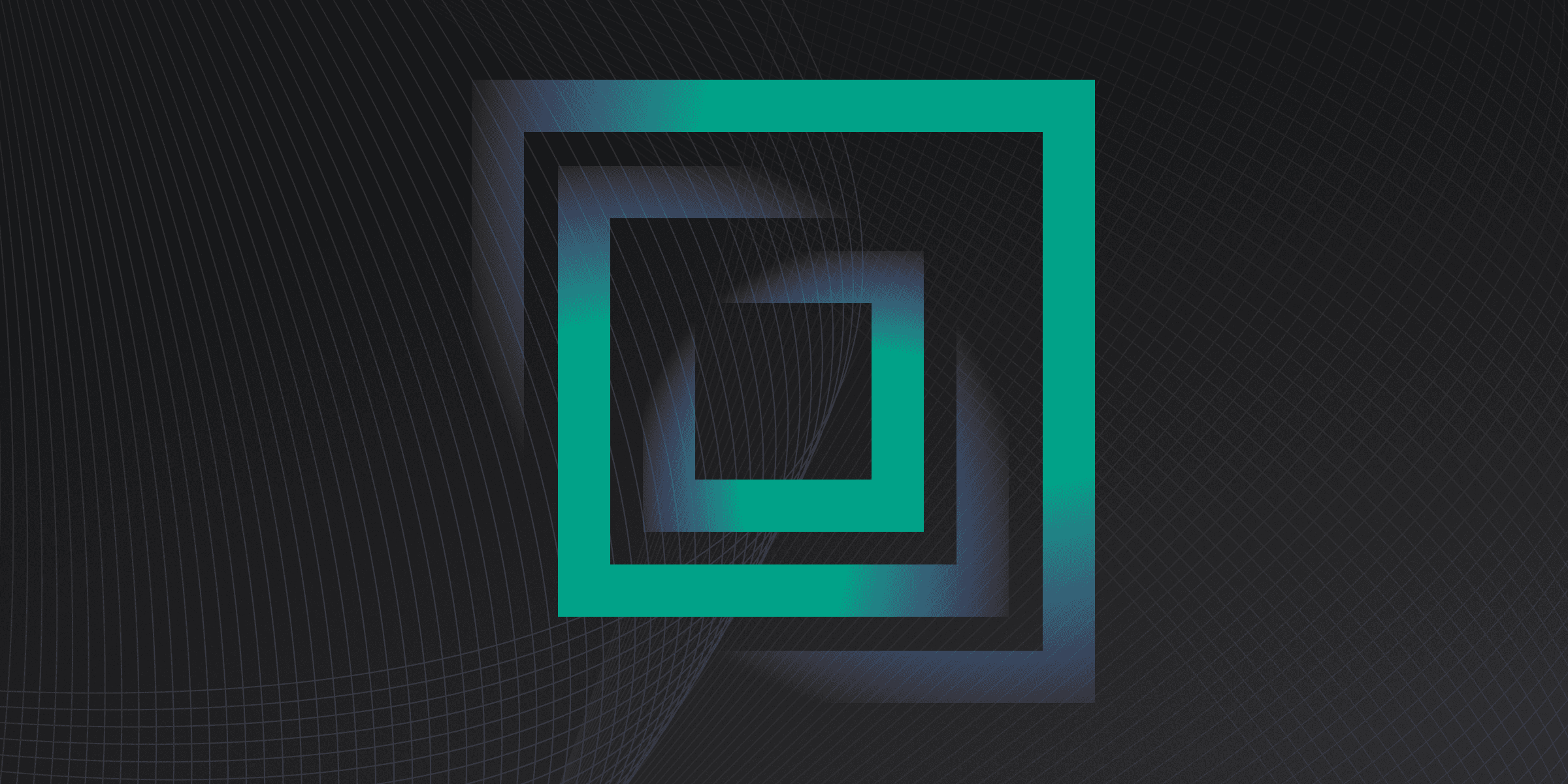
An inference attack is a data mining technique where attackers deduce sensitive information from seemingly unrelated data points.
Types of Inference Attacks
Inference attacks come in various forms, each exploiting different types of data to deduce sensitive information. Understanding these types can help in developing better security measures to protect against them.
Statistical Databases: Attackers analyze statistical data to infer individual records.
IoT Devices: Data from smart devices can reveal personal information about users.
Mobile Devices: Sensor data like accelerometers can infer user behavior and location.
Voice Recordings: Analyzing voice commands to extract sensitive information.
Recognizing Inference Attack Vulnerabilities
Recognizing inference attack vulnerabilities is crucial for safeguarding sensitive information. By understanding the various ways attackers can exploit data, organizations can better protect their assets and user privacy.
Statistical Data: Attackers analyze aggregated data to infer individual details.
IoT Devices: Poorly secured smart devices can leak personal information.
Mobile Sensors: Data from sensors like accelerometers can reveal user behavior.
Voice Data: Analyzing voice recordings to extract sensitive information.
Mitigating Inference Attacks Strategies
Mitigating inference attacks requires a multi-faceted approach to ensure data security and privacy. By implementing robust strategies, organizations can significantly reduce the risk of sensitive information being deduced from seemingly unrelated data points.
Polyinstantiation: Maintain multiple records with the same key to prevent inference.
Data Anonymization: Modify fields while preserving aggregate characteristics.
Defense In-Depth: Use multiple layers of security to guard against failures.
Least Privilege: Allow minimal permissions necessary for tasks.
Separation of Duties: Split privileges among individuals to prevent fraud.
Inference Attacks in the Wild
Inference attacks are not just theoretical; they have been observed in various real-world scenarios, demonstrating their potential to compromise sensitive information. Here are some notable examples of inference attacks in the wild:
Healthcare Data: Attackers deduced patient information from anonymized health records.
Social Media: Personal details inferred from public posts and interactions.
Smart Meters: Energy usage patterns revealing household activities.
Mobile Apps: User behavior inferred from app usage and sensor data.
Voice Assistants: Sensitive information extracted from voice command patterns.
Rapidly implement a modern Zero Trust network that is more secure and maintainable than VPNs.
What is an Inference Attack?
Twingate Team
•
Oct 9, 2024
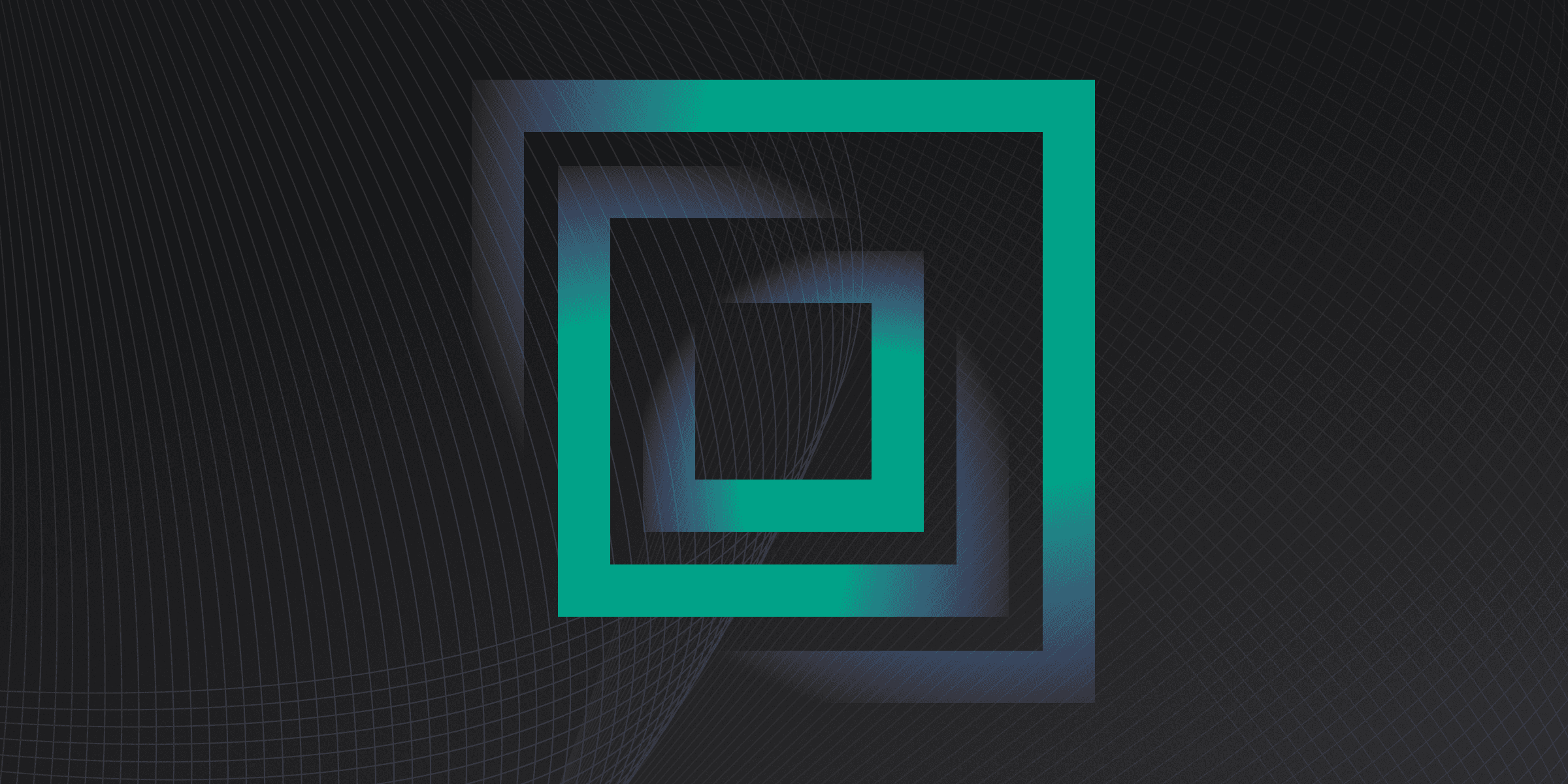
An inference attack is a data mining technique where attackers deduce sensitive information from seemingly unrelated data points.
Types of Inference Attacks
Inference attacks come in various forms, each exploiting different types of data to deduce sensitive information. Understanding these types can help in developing better security measures to protect against them.
Statistical Databases: Attackers analyze statistical data to infer individual records.
IoT Devices: Data from smart devices can reveal personal information about users.
Mobile Devices: Sensor data like accelerometers can infer user behavior and location.
Voice Recordings: Analyzing voice commands to extract sensitive information.
Recognizing Inference Attack Vulnerabilities
Recognizing inference attack vulnerabilities is crucial for safeguarding sensitive information. By understanding the various ways attackers can exploit data, organizations can better protect their assets and user privacy.
Statistical Data: Attackers analyze aggregated data to infer individual details.
IoT Devices: Poorly secured smart devices can leak personal information.
Mobile Sensors: Data from sensors like accelerometers can reveal user behavior.
Voice Data: Analyzing voice recordings to extract sensitive information.
Mitigating Inference Attacks Strategies
Mitigating inference attacks requires a multi-faceted approach to ensure data security and privacy. By implementing robust strategies, organizations can significantly reduce the risk of sensitive information being deduced from seemingly unrelated data points.
Polyinstantiation: Maintain multiple records with the same key to prevent inference.
Data Anonymization: Modify fields while preserving aggregate characteristics.
Defense In-Depth: Use multiple layers of security to guard against failures.
Least Privilege: Allow minimal permissions necessary for tasks.
Separation of Duties: Split privileges among individuals to prevent fraud.
Inference Attacks in the Wild
Inference attacks are not just theoretical; they have been observed in various real-world scenarios, demonstrating their potential to compromise sensitive information. Here are some notable examples of inference attacks in the wild:
Healthcare Data: Attackers deduced patient information from anonymized health records.
Social Media: Personal details inferred from public posts and interactions.
Smart Meters: Energy usage patterns revealing household activities.
Mobile Apps: User behavior inferred from app usage and sensor data.
Voice Assistants: Sensitive information extracted from voice command patterns.
Solutions
Solutions
The VPN replacement your workforce will love.
Solutions